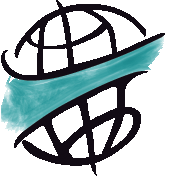 |
Tropentag 2023, September 20 - 22, Berlin, Germany
"Competing pathways for equitable food systems transformation: trade-offs and synergies."
|
Transfer learning for smallholder field delineation and field size estimation in sub-Saharan Africa
Philippe Rufin, Patrick Meyfroidt
UCLouvain, Earth and Life Institute, Belgium
Abstract
Agricultural monitoring based on Earth observation is challenging in tropical smallholder landscapes, due to the spatially fragmented and dynamic nature of the agricultural systems leading to region-specific and diverse portfolios in terms of crops, productivity, and land management. These complexities are particularly prevalent in sub-Saharan Africa, where a large fraction of the agricultural land is managed by semi-subsistence smallholder farmers, which operate under constrained access to capital and inputs. As a consequence, the spatial distribution of agricultural fields, field sizes, crop types, and productivity remains an empirical blind spot for most parts of sub-Saharan Africa.
In this study, we combine state-of-the-art deep learning models with very-high spatial resolution satellite imagery to produce accurate delineations of individual smallholder fields. We make use of transfer learning by fine-tuning deep learning models trained in France and India for use in northern Mozambique and southwest Nigeria. We experiment with different strategies for fine-tuning models across regions based on human-annotated training data and investigate options for self-supervision to facilitate model transfer while reducing reference data requirements. We test the performance of pre-trained and fine-tuned models for object-level field delineation and site-level field size estimation based on the median intersection over union (mIoU) and mean site-level RMSE (mRMSE), respectively using independent reference data.
Our results indicate i) good performance of the pre-trained model in both field delineation (mIoU: 0.699) and field size estimation (mRMSE: 0.071 ha), ii) consistent performance improvements for all fine-tuning experiments compared to the pre-trained model (mIoU increases: 0.045 - 0.058, mRMSE decreases: 0.008 ha - 0.031 ha), revealing substantial improvements achieved by regional fine-tuning, and iii) comparable performance increases for supervised and self-supervised approaches, with low differences in object-level (ΔmIoU: 0.014 ), and site-level performance scores (ΔmRMSE 0.017), indicating a great potential for self-supervised transfer learning in reducing reference data requirements. This study presents a stepping stone for overcoming the persisting data gaps in smallholder agriculture of sub-Saharan Africa by producing detailed field delineations at scale, which support field-level crop type and yield estimation, and allow for field size estimation in smallholder regions.
Keywords: Deep learning, field size, geospatial, India, land management, machine learning, Mozambique, Nigeria, remote sensing, sub-Saharan Africa
Contact Address: Philippe Rufin, UCLouvain, Earth and Life Institute, Place louis pasteur 3, 1348 Louvain-la-neuve, Belgium, e-mail: philippe.rufin uclouvain.be
|