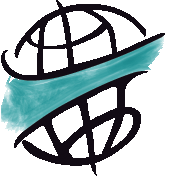 |
Tropentag 2023, September 20 - 22, Berlin, Germany
"Competing pathways for equitable food systems transformation: trade-offs and synergies."
|
Crop yield estimation using mobile pictures and deep learning in collaboration with thousands of smallholder coffee producers
Juan Camilo Rivera Palacio1, Christian Bunn 2, Ryo Masahiro1
1Leibniz-Centre for Agricultural Landscape Research (ZALF), Research Platform Data Analysis and Simulation, Germany
2The Alliance of Bioversity International and CIAT, Climate Action, Colombia
Abstract
The use of earth observation data, unmanned aerial vehicle imagery and manual counting for coffee crop monitoring are popular methods for predicting crop yield in Latin America. However, the coffee crop's environmental conditions, such as systems under shade, along with the time-consuming nature of the process, create a barrier to the extensive use of these methods at plot and larger scales. This study explored the use of mobile pictures of coffee trees for yield prediction at tree level in agroforestry systems for five coffee varieties in Colombia and Peru, in the form of citizen science. The object detection deep learning model, ”You Only Look Once” YOLO v5, and manual counting were used together with extensive field monitoring data comprising information from 977 farmers, 2954 trees, and 10195 mobile pictures. The model was trained with two varieties of trees, Catimor Cogollo Morado and Catimor Cogollo Verde, in Peru. The training data consisted of ca. 35,000 labeled cherries. After model training, we deployed the model in Colombia with varieties variedad Colombia and supremo to test if the model can be useful in other regions. The results of yield prediction revealed an R-squared value of 63%. The model achieves good performance with unseen data, and the model performance was as good as previous studies which employ more advanced but expensive techniques. Our study demonstrated that the method can generate yield predictions in seconds without the need for any expensive monitoring devices. Additionally, the use of mobile pictures can generate yield data for multiple zones where there is a lack of such data. This indicates the possibility of using mobile pictures to predict crop yield at the plot and even much larger scales for efficient planning and access to financial services. Future work should focus on the role of human users in the use of the machine vision system to further improve the accuracy of the results.
Keywords: Artificial intelligence, citizen science, coffee, deep learning, object detection
Contact Address: Juan Camilo Rivera Palacio, Leibniz-Centre for Agricultural Landscape Research (ZALF), Research Platform Data Analysis and Simulation, Müncheberg, Germany, e-mail: juancamilo.rivera zalf.de
|