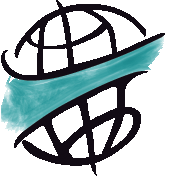 |
Tropentag 2023, September 20 - 22, Berlin, Germany
"Competing pathways for equitable food systems transformation: trade-offs and synergies."
|
Leveraging earth observation and crop modelling for crop condition monitoring and production assessment in Busia County, Kenya
Harison Kipkulei1, Francis Oloo2, Pamela Ochungo2, Stefan Sieber1, Hussein Farah2, Gohar Ghazaryan1
1Leibniz Centre for Agricultural Landscape Research (ZALF), Germany
2The Technical University of Kenya (TUK), School of Surveying and Spatial Science, Kenya
Abstract
Understanding the dynamics of agricultural productivity in highly heterogeneous smallholder landscapes is critical for improving food and livelihood security. This study presents an integration of crop modelling and remote sensing approaches to improve the understanding of crop conditions and assess the productivity of dominant crops in Busia County in Kenya. Effectively, crop modelling and remote sensing analysis using multi-source and freely available earth observation data are proposed to classify the cropping systems in the region, predict yield, and assess the influence of climatic stressors on crop production. The first step applies synthetic aperture radar data from Sentinel-1 and optical imagery from Sentinel-2 to map crop types in the study area. The classification is based on the existing crop type information from different sources, which is harmonised for the study area and is used for pixel-level classification using the random forest machine learning model for the years where the reference data is available. The trained model is later used for the same years when the training data is available and the following year for an early-season crop map. These maps, along with meteorological forecasts, existing drought risk, and yield information, are used to derive essential agro-climatic indicators. The output from the classification process is further integrated into the simulation of maize production and assessment of yield anomaly in the study area for a seven-year period in which observed yield is available. For this, a parameterised and evaluated DSSAT-CERES-Maize model is proposed to predict yields and assess yield anomalies at fine spatial and temporal scales. The simulated dynamics of production across the study region are useful for enhancing agronomic decisions and driving food security planning in the region. The study highlights the value of integrating earth observation data and crop modelling to enhance the understanding of smallholder agricultural productivity. The proposed approach is scalable to other regions and relies on freely available data. Thus, it could improve the monitoring of crop conditions and productivity in data-scarce landscapes.
Keywords: Climatic stressors, crop conditions, phenology, remote sensing
Contact Address: Harison Kipkulei, Leibniz Centre for Agricultural Landscape Research (ZALF), Eberswalderstraße 84, 15374 Müncheberg, Germany, e-mail: harison.kipkulei zalf.de
|