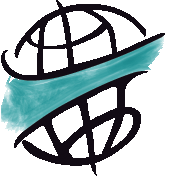 |
Tropentag 2023, September 20 - 22, Berlin, Germany
"Competing pathways for equitable food systems transformation: trade-offs and synergies."
|
Assessing spatio-temporal patterns of rain-fed and irrigated agriculture using time-series earth observation in Baringo, Kenya
Faith Kandie1, Barthlomew Kuria1, Mark Boitt1, Harison Kipkulei2
1Dedan Kimathi University of Technology, Inst. of Geomatics, GIS & Remote Sensing, Kenya
2Leibniz Centre for Agricultural Landscape Research (ZALF), Germany
Abstract
Food security and sustainable agriculture are part of SDG2 (Zero Hunger) and also among the five pillars of the Kenyan government Bottom-Up Economic Plan. Agriculture is the main source of food and with population increase combined with climate variability, transformation to smart agricultural practices arise, one of which is irrigation. Spatial information on existing agricultural land is vital for a framework towards irrigation. Baringo South sub-county is a semi-arid, marginalised and food-insecure area in Kenya with poor coping mechanism towards climate change. The sub-county has an irrigation potential of 180 km2 with 15.8 km2 operational in the large-scale irrigation schemes of Perkerra and Eldume while the small-holder schemes remain unaccounted for hence inadequate statistics on total irrigated land.
This study is thus aimed at assessing the spatial-temporal patterns of rain-fed and irrigated agriculture through: (i) Monitoring existing agricultural land-use employing Landsat time series for1989-2022. (ii) Investigating the extents of irrigated land using Sentinel-2 imagery for 2022 by object-based image analysis (GEOBIA), and (iii) quantifying the importance of irrigated agriculture in food production. Other supplementary data for the study are climate data, vegetation indices and reference data for dominant irrigated, rain-fed lands and other land management practices for training and validation. Objective one results revealed fluctuations in agricultural land with the highest surge and decline of 500 km2 (1989-2002) and 300 km2 (2002-2012), respectively. The changes are attributed to anthropogenic factors causing mutual conversion with rangeland and forests. The highest overall accuracy was 89.09% with a kappa of 0.87. Objectives two and three analysis are ongoing at reference data collection stage. For classification of both Landsat and Sentinel, the robust machine learning Random Forest Algorithm was/will be executed. These results will benefit in transforming food system through revelation of patterns, extents and significance of irrigation in food production.
Keywords: Agriculture, food security, irrigation, Kenya, forest conversion
Contact Address: Faith Kandie, Dedan Kimathi University of Technology, Inst. of Geomatics, GIS & Remote Sensing, Ngumba Drive, 00608 Nairobi, Kenya, e-mail: rjk.faith gmail.com
|