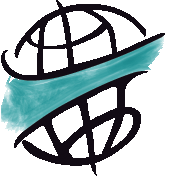 |
Tropentag 2023, September 20 - 22, Berlin, Germany
"Competing pathways for equitable food systems transformation: trade-offs and synergies."
|
Predicting potato diseases in smallholder agricultural areas of Nigeria using machine learning and remote sensing-based climate data
Esther S. Ibrahim1, Claas Nendel1, Bahareh Kamali2, Efron N. Gajere3, Patrick Hostert1
1Leibniz-Centre for Agric. Landscape Res. (ZALF), Germany
2University of Bonn, Inst. Crop Sci. and Res. Conserv. (INRES), Germany
3National Space and Research Development Agency, Space Institude, Nigeria
Abstract
In many regions of Africa, severe cases of pest and crop diseases are linked to the negative impacts of climate change such as rising average temperatures and changes in precipitation regimes. The problems are prominent in Nigeria, where rainfed subsistence farming dominates and even only slight changes in climate regimes may largely affect cropping systems. Although farmers in Nigeria continue to use broad-spectrum fungicides on potato, potato diseases are still on the rise. Using remote sensing data and modeling techniques, related risks may be mitigated in the future, but a deep understanding of the mechanisms behind crop diseases or operational early-warning systems is not in place in Nigeria and most parts of SSA. Recently, machine-learning methods have become more prominent in epidemiological early warning. They provide vital information on data-disease relations useful for pre-disease infection management. Here, we build on machine-learning methods to develop spatial early warning tools for Nigeria, using the Jos Plateau as a test case. Remote sensing, as well as meteorological and field data, were used to (a) predict disease incidences using field reference data and a random forest classifier (RF), and (b) identify local conditions conducive to potato diseases, using machine-learning results based on multi-criteria classification (MCC). The results of the RF approach for 2019, 2020, and 2021 showed similar spatial characteristics, while the MCC varied significantly. Both models predicted between 72 % and 96 % of the potato fields being infested. The MCC model further revealed that spatiotemporal frequencies of vulnerability in June can be the indicator that informs the degrees of infestation. RF predictions resulted in higher levels of accuracy (between 83 % and 88 %) compared to MCC (between 67 % and 77 %), while the latter reflected the spatiotemporal patterns of annual disease incidence better. A five-day vulnerability window used with the MCC proved to be the most useful tool for developing an efficient spraying regime, based on a combination of temperature, rainfall, and relative humidity thresholds. This facilitates our development of an operational early-warning system for potato disease in tropical highlands of Africa, especially introducing spatial risks, which provides a more eco-friendly approach for early warning. This supports future smart agricultural practices and food security, increases yields, and promotes sustainable agricultural policies.
Keywords: Classification, epidemiology, modelling, prediction, random forest, spatial sensing
Contact Address: Esther S. Ibrahim, Leibniz-Centre for Agric. Landscape Res. (ZALF), Eberswalder Straße 84 , 10099 Müncheberg, Germany, e-mail: esther_shupel.ibrahim zalf.de
|