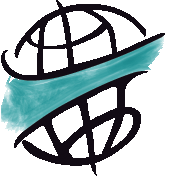 |
Tropentag 2023, September 20 - 22, Berlin, Germany
"Competing pathways for equitable food systems transformation: trade-offs and synergies."
|
Combining crop-cuts with remotely-sensed weather data to predict maize yields in sub-Saharan Africa
Max Hofmann1,2, Lena Büschel2,1, Florian Schierhorn1, Christopher Krause1,2, Daniel Müller3,1
1IAMO - Leibniz Institute of Agricultural Development in Transition Economies , Structural Change, Germany
2Martin Luther University Halle-Wittenberg, Germany
3Humboldt-Universität zu Berlin, Integrative Research Institute on Transformations of Human-Environment Systems (IRI THESys), Germany
Abstract
Weather extremes increasingly threaten agriculture and food security, particularly in the Global South. In sub-Saharan Africa, where agriculture is dominated by smallholder farming, extreme events such as droughts and heavy rainfall frequently lead to production shortfalls that jeopardise local livelihoods. We evaluated how weather patterns determine yield variability using 20,660 maize yield measurements recorded between 2016 and 2020 from six countries (Kenya, Tanzania, Rwanda, Malawi, Zambia, Burundi). These so-called crop-cuts are considered the gold standard for yield estimation because they reflect actual plot-level harvests that are weighed and recorded according to standardised protocols. We used the dates for seeding and harvest of each crop-cut to specify the length of the growing season and calculated extreme weather indices for each crop-cut-specific growing season from freely available remote sensing products, including drought, heat, heavy precipitation, and evaporative stress. We used random forests, a machine learning algorithm that can handle collinearity among predictor variables and nonlinear relationships with the response variable, to quantify the contribution of weather to maize yields. Depending on the country, our out-of-sample predictions reach R2-values of between 0.14 and 0.42, suggesting that weather explains up to almost half of the observed yield variability in some regions. This study advances the insights into assessing the effects of extreme weather on smallholder yields with machine learning. Our results can inform the development of tailored adaptation measures such as local index insurance products and ultimately contribute to improving regional food security in light of accelerating climate change and increasing risk for future extreme weather events.
Keywords: Climate change, crop-cuts, extreme weather, machine learning, yield prediction
Contact Address: Max Hofmann, IAMO - Leibniz Institute of Agricultural Development in Transition Economies , Structural Change, Theodor-Lieser-Str. 2, 06120 Halle (Saale), Germany, e-mail: hofmann iamo.de
|