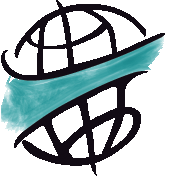 |
Tropentag 2023, September 20 - 22, Berlin, Germany
"Competing pathways for equitable food systems transformation: trade-offs and synergies."
|
Mapping heterogeneous smallholder maize-cassava systems in southwest Nigeria based on Sentinel-1 and Sentinel-2 time series
Felicia O. Akinyemi1, Philippe Rufin2, Esther S. Ibrahim3, Patrick Hostert4, Lucia O Ogunsumi5, Olugbenga A. Egbetokun5, Chinwe Ifejika Speranza1
1University of Bern, Inst. of Geography - Land Systems and Sustainable Land Management, Switzerland
2UCLouvain, Earth and Life Institute, Belgium
3Leibniz-Centre for Agric. Landscape Res. (ZALF), Germany
4Humboldt-Universität zu Berlin, Geography Department, Germany
5Obafemi Awolowo University, Inst. of Agricultural Research and Training, Nigeria
Abstract
Earth observation is key for agricultural monitoring across large regions. Operational agricultural monitoring schemes involve the mapping of agricultural inputs, land management and/or crop types. Approaches developed in consolidated agricultural systems in Europe or the United States, have limited applicability in more heterogeneous systems. An example is crop type mapping which is challenged by the presence of inter-cropping, i.e., the simultaneous presence of multiple crops on a field, due to the high intra-field variability in crop types, growth stages and non-crop vegetation. Consequently, regional-scale crop type mapping approaches in smallholder agriculture mostly focus on specific crops and do not account for crop mixtures or double growing cycles.
A combination of Sentinel-1 radar and multispectral Sentinel-2 time series was used to separate mono-cropping from mixed-cropping in heterogeneous maize-cassava farming systems across the lower Guinea Savannah of southwest Nigeria. The region is characterised by high cloud cover during much of the growing season that affects non-cloud-penetrating Sentinel-2 optical data, while it provides much richer spectral information than Sentinel-1 radar data. Field data for the growing season 2022/2023 comprise ~1,150 samples. Eight classes were defined indicating crop types, mixtures, and growing periods (early maize, late maize, early cassava, late cassava, maize-cassava, yam, rice, and other crops). Gap-free spectral-temporal features at bi-weekly, monthly, and bi-monthly intervals for the growing season (Sentinel-1) and the off-season (Sentinel-2) were pro-cessed in Google Earth Engine. Random Forest models were trained to conduct experiments assessing the suitability of the two sensors and the three temporal intervals. Results were validated using k-fold cross-validation.
The best model combining Sentinel-1 and Sentinel-2 at monthly intervals yielded a mean overall accuracy of 0.78 (+/-0.08%) and median User´s and Producer´s accuracies above 0.60 for all classes. Models using only radar data underperformed with mean overall accuracies below 0.50 and models using only multispectral data had comparable overall accuracies but lower class accuracies for the mixed classes. Our approach demonstrates the potential of integrating radar and optical time series in cloud-prone regions with complex agricultural and forest mosaic landscapes involving mixed crops and thereby provides valuable information for downstream assessments of crop production and yields.
Keywords: Crop mixtures, crop type mapping, maize-cassava, Random Forest, Sentinel-1, Sentinel-2, smallholder farming systems, spectral-temporal metrics
Contact Address: Felicia O. Akinyemi, University of Bern, Inst. of Geography - Land Systems and Sustainable Land Management, Hallerstrasse 12, 3012 Bern, Switzerland, e-mail: felicia.akinyemi gmail.com
|