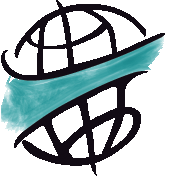 |
Tropentag 2021, September 15 - 17, hybrid conference, Germany
"Towards shifting paradigms in agriculture for a healthy and sustainable future"
|
CO2 Dynamics in the Pore Volume of Stored-Grain Bulk: Data Acquisition and Analysis via Machine Learning
Iris Ramaj, Steffen Schock, Joachim Müller
University of Hohenheim, Inst. of Agricultural Engineering, Tropics and Subtropics Group , Germany
Abstract
Quality preservation is one of the major considerations in the conception and operation of grain storage facilities. Warm and humid conditions have a direct impact on the temperature and moisture changes of the grain mass beyond the safe-storage thresholds. Consequently, favourable conditions for the development of microorganisms, fungi and toxic contaminants can be created, leading to elevated CO2 concentrations in localized pockets of the grain mass. In this regard, real-time monitoring can be an important tool to control incipient grain deterioration. Thus, this study aimed to monitor the grain storage conditions in real-time and investigate their influence on the intergranular CO2 dynamics. Variables considered in the analysis were temperature, relative humidity, moisture content, atmospheric pressure, and height of grain bulk. For the data acquisition, a small-scale silo (2011 mm diameter and 5040 mm height) filled with freshly harvested wheat grains (Pioneer A, DSV AG) at a moisture content of 0.16 kg kg-1 dry basis was used. A sensor system network with linear topology comprised of 12 multifunctional sensor nodes designed in-house were installed equidistantly within the bulk to monitor the temporal and spatial variations of grain conditions. As an analytical approach, supervised machine learning using Gaussian process regression (GPR) was employed. A dataset of 360 consecutive days of grain storage with internment cooling and measuring resolution of 10 min was selected for the training and validation procedures. The dynamics of CO2 within the grain bulk were found to be strongly influenced by the diurnal and seasonal changes of the external environmental conditions. Results exhibited significant interactions among the variables analysed. An increase of CO2 was observed with the increase of temperature, relative humidity and moisture content of in-store grain. In contrast, the levels of intergranular CO2 decreased as the atmospheric pressure and bulk height increased. The applied machine learning approach yielded a highly accurate prediction (R2 = 0.99 and RMSE = 20.65 ppm), demonstrating its great potential to accurately predict the dynamics of CO2 depending on the temporal and spatial changes of in-store grain conditions.
Keywords: CO2, grain storage, machine learning, monitoring, sensors
Contact Address: Iris Ramaj, University of Hohenheim, Inst. of Agricultural Engineering, Tropics and Subtropics Group , Garbenstr. 9, 70599 Stuttgart, Germany, e-mail: Ramaj uni-hohenheim.de
|