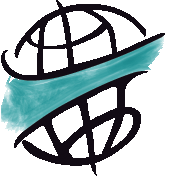 |
Tropentag, September 18 - 20, 2019 in Kassel
"Filling gaps and removing traps for sustainable resources development"
|
Using Crowdsourcing and Machine Learning for Predicting the Spatial Distribution of Banana-Based Cropping Systems in Uganda
Dennis Ochola1, Godfrey Taulya2, Gerrie van de Ven1, Ken Giller1
1Wageningen University & Research, Plant Production Systems Group, The Netherlands
2International Institute of Tropical Agriculture (IITA), Uganda
Abstract
Uganda is the leading global producer of highland bananas (Musa spp. AAA) endemic to East Africa. For decades, expert opinion has been the source of information on the spatial distribution of banana-based cropping systems in Uganda. Lack of accurate and reliable spatial data undermines strategic planning and sustainable intensification at various scales. This study uses 18,956 crowdsourced presence-absence data coupled with geospatial data from 71 covariates (21 climatic, 19 edaphic, 19 vegetation, 6 topographic and 6 socio-economic) to predict the spatial distribution of banana-based cropping systems using the machine learning algorithms Random Forests (RF), Gradient Boosting Machines (GBM) and Neural Networks (NNET). Performance of RF and GBM was better than NNET in terms of accuracy, receiver operating characteristic (ROC) and sensitivity. But, NNET performed better with regards to Cohen's kappa and specificity. The ensemble model aggregating outcomes of RF, GBM and NNET performed better (AUC = 0.881) compared to the logistic regression model (AUC = 0.852). Spatial predictions revealed that banana-based cropping systems occupied 9.6% of the total land area of Uganda. The probability of banana presence was greater (>0.6) in the western (i.e. Ankole, Toro and foothills of Mt Rwenzori), central (i.e. Buganda in Kooki and Buddu) and eastern (i.e. foothills of Mt Elgon), and least (<0.2) in the northern region. Geographic shifts are defined by declines in the eastern (-13.4%), stagnation in the central (-4.3%) and expansion in the western (+17.3%). Although machine learning can iteratively search and filter through covariates to achieve high prediction accuracy, including redundant covariates in the best-fit model may not explicitly describe prediction outcomes. Thus, hypothesis-based selection of covariates with known influence on banana growth and agronomic management is a better option for identifying the drivers of geographic shifts of banana-based cropping systems in Uganda.
Keywords: Banana, banana-based cropping systems, crowdsourcing, geographical shift, machine learning, Uganda
Contact Address: Dennis Ochola, Wageningen University & Research, Plant Production Systems Group, Wageningen, The Netherlands, e-mail: dennis.ochola wur.nl
|