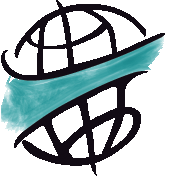 |
Tropentag, September 18 - 20, 2019 in Kassel
"Filling gaps and removing traps for sustainable resources development"
|
Agronomic Intelligence: Data Management, Modelling and ICT to Deliver Site-Specific Recommendations to Smallholder Farmers
Meklit Chernet Tariku1, Kodjovi Senam Ezui2, Christine Kreye3, Stefan Hauser3, Theresa Ampadu-Boakye2, Veronica N.E. Uzokwe4, Bernard Vanlauwe1, Pieter Pypers1
1International Institute of Tropical Agriculture (IITA), Kenya
2African Plant Nutrition Institute, African Cassava Agronomy Initiative, Kenya
3International Institute of Tropical Agriculture (IITA), Nigeria
4International Institute of Tropical Agriculture (IITA), Tanzania
Abstract
Blanket recommendations for agronomic interventions often bring along substantial risks for smallholder farmers, as responses vary due to heterogeneity in soil conditions, weather, management practices and input-output prices. By considering this heterogeneity, however, site-specific recommendations can be delivered that maximise the profitability and reduce the risk of investments in these technologies. Developing site-specific recommendations at scale requires identifying best agronomic interventions, testing how these perform in field experiments across conditions within the target intervention area, and then using advanced data analysis techniques and crop models to predict crop responses to these interventions. This is then packaged in a convenient format as decision support tools (DSTs) to deliver recommendations to extension agents and farmers. The African Cassava Agronomy Initiative (ACAI) has developed tailored recommendations for cassava growers in Nigeria and Tanzania, responding to demands identified by stakeholders in the cassava value chain. To overcome the limitations of conventional agronomy research, ACAI developed a smart agronomy data management system (SandMan) by combining barcode identifiers and digital data collection forms, a cloud-based server to host data centrally and R scripts for data curation and analysis. This approach allowed managing thousands of field trials and assess the impact of the environment on the efficacy of agronomic interventions. Prediction models were developed to relate responses to tillage, cassava plant density, fertiliser application, intercropping and varied planting and harvest dates to GIS soil and weather layers using a combination of crop models and machine learning techniques. Field-scale recommendations are then generated accounting for crop produce and fertiliser prices, the farmer's investment capacity and risk attitude. Recommendations are packaged in diverse formats tailored to the dissemination strategies of last-mile delivery partners. DST formats include paper guides and maps, as well as electronic tools: mobile apps, IVR, USSD and SMS. Currently, a pilot study involving over 2,000 households and 200 extension agents is ongoing to evaluate the accuracy and return-on-investment of the recommendations, and the user experience of the various formats to anticipate the reach and impact of the recommendations delivered.
Keywords: African Cassava Agronomy Initiative (ACAI), crop models, decision support tools , digital data collection
Contact Address: Meklit Chernet Tariku, International Institute of Tropical Agriculture (IITA), Nairobi, Kenya, e-mail: m.chernet cgiar.org
|