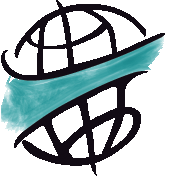 |
Tropentag, September 20 - 22, 2017 in Bonn
"Future Agriculture: Social-ecological transitions and bio-cultural shifts"
|
Crop Type and Condition Monitoring: A Multi-Temporal and Multi-Sensor Approach
Gohar Ghazaryan1,2, Olena Dubovyk2, Fabian Löw3, Nataliia Kussul4, Jürgen Schellberg5
1University of Bonn, Center for Development Research (ZEF), Germany
2University of Bonn, Center for Remote Sensing of Land Surfaces (ZFL), Germany
3Maptailor Geospatial Consulting GbR, Germany
4Space Research Institute of National Academy of Sciences of Ukraine and State Space Agency of Ukraine, Ukraine
5University of Bonn, Inst. Crop Sci. and Res. Conserv. (INRES), Germany
Abstract
Due to growing population, sparse land and water resources, the need for enhancing agricultural productivity to ensure food security is increasing. Accurate crop maps and other data products derived from earth observation can build the basis for agricultural monitoring at different ranges of scale. Such data are one of the essential means to support sustainable land management. In this study, we exploited the intra-annual temporal signatures of remotely sensed observations and used the prior knowledge of crop calendars to create a sequential processing chain for crop classification and condition monitoring by focusing on drought impact.
Time series observations that were derived from both optical (Landsat-8, Sentinel-2) and Synthetic aperture radar (SAR) sensors, were used in order to identify the crop type and productivity reduction due to moisture scarcity or increased evaporative demand.
Landsat-based time-series metrics that capture the within season phenological variations were preprocessed and analysed using Google Earth Engine cloud computing platform. The development stage of each crop throughout the growing season was modeled and the model's output was further used for the automatic generation of training samples. Sentinel-1 images were used as additional input of contextual feature information to classification. Two classification schemes were applied (Random forest and decision fusion) to discriminate the main crops in the study area: cereals (wheat, barley), maize, soy, and sunflower. The Normalized Difference Vegetation Index (NDVI) and dual (VV + VH) polarisation backscattering time series were used to identify the main characteristics of drought (start, end, duration, intensity) for different crops during different stages of the crop development.Tested methods yielded acceptable levels of accuracies in the range of 80-86 %. Combined use of the Landsat time series and Sentinel-1 data improved the classification accuracy. These sensors offer imagery with 10-20m to 30m spatial resolution, providing opportunities for regional monitoring of crop conditions.The methods were tested on data from two consecutive years, which enabled us to study the effect of inter-annual meteorological differences. Based on our results, we recommend the use of multimodal satellite data to derive accurate crop maps and information on crop condition over several years.
Keywords: Crop mapping, drought hazard monitoring, Landsat, Random forest, Sentinel-1
Contact Address: Gohar Ghazaryan, University of Bonn, Center for Development Research (ZEF), Genscherallee 3, 53113 Bonn, Germany, e-mail: gghazary uni-bonn.de
|