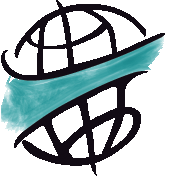 |
Tropentag, September 19 - 21, 2016 in Vienna, Austria
"Solidarity in a competing world - fair use of resources"
|
Towards Improved Land-Use Mapping of Irrigated Croplands: Evaluation of Field- and Pixel-based Parametric and Non-Parametric Image Classification Algorithms
Amit Kumar Basukala1, Carsten Oldenburg1, Jürgen Schellberg2, Gunter Menz3, Murodjan Sultanov4, Olena Dubovyk2
1University of Bonn, Centre for Remote Sensing of Land Surfaces (ZFL), Germany
2University of Bonn, Inst. Crop Sci. and Res. Conserv. (INRES), Germany
3University of Bonn, Dept. of Geography, Remote Sensing Research Group, Germany
4Urgench State University, Uzbekistan
Abstract
An accurate agricultural land use map is an essential input for many agro-environmental applications such as crop land and water management. Enhancement of the accuracy of remote sensing based land use maps is still an ongoing process, since the development of the first classification algorithms for satellite datasets in the 1970s. With the rapid advances in computer technology, Earth Observation sensors and geographical information system (GIS), the field based (FB) and object based (OB) image analysis evolved along with the development of the machine learning algorithms. Studies showed that regardless of availability of different classification methods and algorithms no particular method has universal applicability and acceptability. This study aimed to compare different classification methods to provide recommendation on the choice of the suitable classification method for improved agriculture land use mapping of irrigated croplands. The comparison is made using two robust non-parametric machine learning algorithms, random forest (RF) and support vector machine (SVM), and a classical parametric algorithm, maximum likelihood (MLC) based on the multi-temporal Landsat 8 OLI imagery. Accuracy assessment showed a significant higher overall accuracy (OA) of the machine learning FB-RF algorithm (87.69%) and FB-SVM algorithm (89.23%) over the PB-RF algorithm (78.28%), PB-SVM (79.23%) and PB- MLC (78.51%). The lowest OA occurred with the FB-MLC (66.87%). The kappa accuracy (KA) is also significantly higher for the FB parametric machine learning FB-RF algorithm and FB-SVM algorithm over the FB MLC and all PB algorithms based classifications. The FB-RF produced visually appealing real agricultural land use map of the area. Based on these results, we recommend the use of the FB based machine learning robust non-parametric algorithms for extracting land use information from satellite imagery captured over spatially heterogeneous irrigated croplands and imply them for further sustainable agro-environmental applications.
Keywords: Crop mapping, Landsat, maximum likelihood, random forest, support vector machine, Uzbekistan
Contact Address: Amit Kumar Basukala, University of Bonn, Centre for Remote Sensing of Land Surfaces (ZFL), Sebastian Str. 39, Bonn, Germany, e-mail: pblkamit gmail.com
|